31 August, 2024
Evonik expands its formulation capabilities for lipid nanoparticles !
Evonik is collaborating with KNAUER Wissenschaftliche Geräte GmbH, a manufacturer of scientific instruments, to improve the upscaling of lipid nanoparticle (LNP) formulations. By combining Evonik’s formulation and scale-up expertise with KNAUER’s technological know-how, customers can improve efficiency and increase speed to market, significantly cutting the initial pre-clinical development time.
As part of the company’s Nutrition & Care division, Evonik’s Health Care business is leveraging partnerships with life science and industry leaders to expand its portfolio of biosolutions, including innovations in nucleic acid-based medicines.
“Our extensive expertise in chemistry and formulation science has enabled us to capture a significant proportion of the growing market for mRNA and gene therapies. Thanks to our collaboration with KNAUER, we are now even better positioned to offer differentiation options,” said Yann d'Hervé, head of the Health Care business line at Evonik.
Nucleic acid therapeutics represent a huge and expanding proportion of the overall parental drugs market. Evonik supplies pharmaceutical companies worldwide with lipids and LNP drug product development activities. The LNP market alone is estimated to be over US$600 million (up to US$1billion) with continued growth projected over the next decade.
"Together with KNAUER, we can increase the speed with which we go from very small-scale prototypes to production-scale lead formulations, and then directly into GMP production of drug products,” said Andrea Engel, head of Growth Projects Evonik Health Care.
KNAUER is known for its high-end scientific instruments used in research and commercial applications. Recently, Evonik implemented KNAUER’s IJM NanoScaler Pro technology, which addresses the long-standing challenges in nucleic acid LNP formulations by reducing the need for labor-intensive and time-consuming experiments, thereby significantly reducing the time and material costs associated with screening for optimal LNP formulations on platforms that do not necessarily upscale when introduced into more proven IJM large scale systems.
"By working hand-in-hand with Evonik, we are confident that our areas of expertise complement each other, and that exceptional formulation and technology will lead to improved patient outcomes," said Anja Fuss, head of Purification & Customized Solutions at KNAUER.
#FormulationScience #ProductDevelopment #Pharmaceuticals #Cosmetics #FoodScience #ChemicalEngineering #DrugDelivery #SkincareFormulation #FlavorScience #Agrochemicals #InnovationInScience #FormulationChemistry #IndustrialFormulation #StabilityTesting #IngredientInteractions
Website: International Research Data Analysis Excellence Awards
Visit Our Website : researchdataanalysis.com
Nomination Link : researchdataanalysis.com/award-nomination
Registration Link : researchdataanalysis.com/award-registration
member ling : researchdataanalysis.com/conference-abstract-submission
Awards-Winners : researchdataanalysis.com/awards-winners
Contact us : contact@researchdataanalysis.com
Get Connected Here:
==================
Facebook : www.facebook.com/profile.php?id=61550609841317
Twitter : twitter.com/Dataanalys57236
Pinterest : in.pinterest.com/dataanalysisconference
Blog : dataanalysisconference.blogspot.com
Instagram : www.instagram.com/eleen_marissa
30 August, 2024
biomedical research !
Biomedical research is a broad field dedicated to understanding health and disease at a fundamental level, with the aim of improving medical treatments, diagnostics, and overall patient care. This area of research spans various disciplines, including biology, chemistry, physics, and medicine.
Here are some key aspects of biomedical research:
- Basic Research: This involves exploring the fundamental mechanisms of living organisms, such as how cells function or how genes are regulated. It provides the foundational knowledge needed to understand disease processes and potential treatments.
Translational Research: This type of research aims to translate discoveries from basic science into practical applications, such as new therapies or diagnostic tools. It's often described as "bench-to-bedside" research, bridging the gap between laboratory findings and clinical applications.
Clinical Research: This research involves testing new treatments, drugs, or interventions in humans. Clinical trials are a crucial part of this phase, assessing the safety and efficacy of new therapies before they become widely available.
Epidemiology: This branch of biomedical research focuses on studying patterns, causes, and effects of health and disease conditions in populations. It helps identify risk factors and inform public health policies.
Genomics and Proteomics: These fields study the genome (complete set of genes) and proteome (complete set of proteins) of organisms. Understanding genetic and protein variations can lead to personalized medicine and targeted therapies.
Biomedical Engineering: This interdisciplinary field combines principles from engineering and biology to develop medical devices, diagnostic tools, and technologies that improve healthcare.
Biomedical research is constantly evolving with advances in technology and methodology, leading to new discoveries and innovations in the medical field. Whether it's through understanding disease mechanisms, developing new treatments, or improving diagnostic techniques, the goal is to enhance human health and quality of life.
Website: International Research Data Analysis Excellence AwardsVisit Our Website : researchdataanalysis.com
Nomination Link : researchdataanalysis.com/award-nomination
Registration Link : researchdataanalysis.com/award-registration
member ling : researchdataanalysis.com/conference-abstract-submission
Awards-Winners : researchdataanalysis.com/awards-winners
Contact us : contact@researchdataanalysis.com
Get Connected Here:
==================
Facebook : www.facebook.com/profile.php?id=61550609841317
Twitter : twitter.com/Dataanalys57236
Pinterest : in.pinterest.com/dataanalysisconference
Blog : dataanalysisconference.blogspot.com
Instagram : www.instagram.com/eleen_marissa
29 August, 2024
Research collaboration: Cross-disciplinary training in sustainable chemistry and chemical processes !
Greg M. Swain, Professor of Chemistry in the Department of Chemistry at Michigan State University, is researching cross-disciplinary training in sustainable chemistry and chemical processes. Here, he emphasizes the importance of teamwork for effective research collaborations
The Research Experiences for Undergraduates (REU) program in the Department of Chemistry at Michigan State University aims to educate students majoring in chemistry, biochemistry, and chemical engineering about significant societal sustainability challenges. The program provides graduate-level interdisciplinary research experiences that address various aspects of these challenges.
The Research Experiences for Undergraduates program
The REU program exposes students to how sustainable practices are impacting research and technology development in chemistry and chemical engineering. The ten-week summer program introduces students, some of whom are engaging in graduate-level research for the first time, to the steps in the research process:Formulation of a research question and or a hypothesis.
Creation of a research design.
Execution of an experimental plan.
Analysis and interpretation of the resulting data.
Reporting on the findings and their significance.
In the U.S., the National Science Foundation (NSF) funds research opportunities for undergraduate students through its REU Sites program. Our program is in its tenth year and has provided education, training, and professional skills development to over 100 individuals from across the contiguous mainland and Puerto Rico. The program’s core goals are:To involve undergraduate students in graduate-level research projects geared toward addressing green and sustainable challenges through an interdisciplinary approach.
To provide a positive mentoring experience and educate the student participants on what constitutes an appropriate and effective mentor-mentee relationship.
To generate interest in and better prepare undergraduate students for success in graduate school.
To produce a research toolbox (lab safety, notebook, basic statistics, communication, team skills) for the students, and
To significantly enhance their professional development, confidence, and self-esteem.
In prior articles, the approach our REU program uses to educate undergraduate students in responsible and ethical conduct of research (1) and to inform them about the importance of mentoring and what their responsibilities are in the mentor-mentee relationship (2) were described.
Additionally, the impact of the independent research experience on a student’s personal and professional growth was discussed (3). In this article, the importance of teamwork for effective research collaborations and how our REU program introduces undergraduate students with limited research experience to the skills needed to be an effective contributor to a research team is recounted.
Collaborations among researchers
Collaborations among researchers across disciplinary, organizational, and cultural boundaries are vital to address increasingly complex societal challenges and opportunities in science and technology development (4,5). Solutions to complex problems in health, the environment, energy, and natural resources require the combined expertise of researchers from different disciplines working effectively and productively together in integrated research teams (6).
Modern-day scientific problems are multifaceted and cannot be adequately addressed by a single discipline. Integrated research teams bring together diverse expertise, allowing for more comprehensive approaches to complex issues and more successful outcomes. Social science data indicates that appropriately applied team training (i.e., team science) positively impacts team performance and innovation (6,7). Training efforts focusing on knowledge, skills, and abilities that are content-appropriate can result in positive team outcomes (8,9).
Effective research collaboration competencies
There are several core competencies needed within teams for effective research collaborations to evolve. These include team communication, effective management of team research tasks, collaborative problem-solving, and overall team leadership (5-7,9). With the increased emphasis on enhancing team outcomes, substantial effort in the social science community has been invested in comprehensive reviews and meta-analyses that have identified core competencies (i.e., knowledge, skills, abilities, and attitudes) that are needed to advance team performance (4-6).
Of course, at a foundational level, the competencies of the individual members determine the effectiveness of the team and the quality of the research collaboration. Students often have the misperception that they can simply be placed within a team and engage in team science. Instead, students must learn and practice specific skills to be effective team members. Our REU program teaches students about some of these important skills and how to practice them so that they become
engrained behaviors.
Team member skills
The program holds weekly one-hour discussions with the REU student participants, during which team skills are the focus. The skills highlighted include:Team members participate willingly, efficaciously, and cooperatively on team assignments and projects.
Team members learn how to identify areas of personal expertise (self- evaluation) and to seek out opportunities to lend expertise to the team to maximize outcomes.
Team members solicit and value input from other team members.
Team members promote an inclusive and equitable working atmosphere through their words and actions to foster collaborative efforts.
Team members listen actively to constructive feedback and during conflict management.
Team members are dependable and follow up on action items that incorporate suggestions to achieve collective objectives.
Teamwork in effective research collaborations
In summary, good teamwork in effective research collaborations requires that the team be guided by effective leadership and management organization and that the team members have appropriate ethical awareness, virtues, and attitudes that are conducive to growth and development. A starting point is educating students with the knowledge, skills, and abilities needed for positive team outcomes.
Visit Our Website : researchdataanalysis.com
Nomination Link : researchdataanalysis.com/award-nomination
Registration Link : researchdataanalysis.com/award-registration
member ling : researchdataanalysis.com/conference-abstract-submission
Awards-Winners : researchdataanalysis.com/awards-winners
Contact us : contact@researchdataanalysis.com
Get Connected Here:
==================
Facebook : www.facebook.com/profile.php?id=61550609841317
Twitter : twitter.com/Dataanalys57236
Pinterest : in.pinterest.com/dataanalysisconference
Blog : dataanalysisconference.blogspot.com
Instagram : www.instagram.com/eleen_marissa
28 August, 2024
RIT offers new master’s degrees in chemical engineering, biomedical engineering, and project management !
RIT has added three new master’s degrees to its portfolio: chemical engineering, biomedical engineering, and project management. Students pursuing graduate degrees are able to combine advanced coursework with hands-on opportunities in laboratories alongside faculty-researchers.
RIT is offering three new master’s degrees designed to meet industry needs.
Chemical engineering and biomedical engineering programs in the Kate Gleason College of Engineering will include new master’s degrees as part of the engineering portfolio this year to meet demands in increasing renewable energies, personalized healthcare technologies, and diagnostic system improvements.
National trends indicate a growing need for graduates with the combined skills in engineering and in the chemical and biological sciences, engineering processes, and ‘smart’ technologies.
The graduate programs will have a mix of students from the established undergraduate programs, as well as new-to-RIT students from regional, national, and international chemical engineering programs seeking advanced degrees. With the flexibility of the degree program, the department also is seeing interest and enrollments from students from other science disciplines such as physics, said Patricia Taboada-Serrano, Graduate Programs Director.
“This will be achieved through a bridge program designed to provide the appropriate engineering background required for successful completion of an advanced degree in chemical engineering,” she said.
A dozen students have been accepted for the new program and will begin chemical engineering courses this fall. There are also eight BS/MS students enrolled in the program who are completing undergraduate work.
There will be several emphasis areas: chemical and mechanical engineering applications; microelectronic focus on semiconductors, photovoltaics, microfabrication; microsystems and quantum level systems; materials science; and advanced mathematics and simulation.
“The strength of our program is the design of its curriculum, as we are able to provide depth in content and advanced skills in one year of studies in the case of full-time students,” said Taboada-Serrano, associate professor of chemical engineering. “The timeline of the completion of the graduate degree enables our MS graduates to rejoin the workforce quickly if they delayed or interrupted careers to obtain a graduate degree. The compactness of our curriculum also enables working professionals to pursue our MS degree and complete it in two to three years.”
Similar to chemical engineering, the biomedical engineering program has grown substantially since it began 10 years ago. Today, 15 students in biomedical engineering (BME) are being integrated into graduate study through the BS/MS options. There are five new students in the stand alone master's program. It is a one-year, course-based program that features a Capstone design sequence.
Biomedical engineers combine knowledge of engineering with biology, anatomy, and physiology to create devices and systems to address the need for sophisticated diagnostic and therapeutic equipment and solutions.
In addition to the advanced engineering degrees, 10 RIT students this semester are the first to enroll in classes for a project management master’s degree.
The 30-credit degree is approved for both in-person and online delivery.
Project management is a process for managing the successful execution of new initiatives within an organization for the sake of expanding the breadth of capabilities, services, and products offered.
“You can use this discipline in almost any field,” said Peter Boyd, senior lecturer and graduate programs director for RIT’s School of Individualized Study, which is overseeing the program. “It’s akin to software engineering in that you could work in numerous industries, from IT to construction to aviation or health care.”
“Project management is a growing discipline. There’s a growing demand in a wide range of industries,” Boyd said.
A RITx MicroMasters in Project Management, offered by SOIS on the edX.org platform, is an additional pathway into the program that allows students to earn RIT course credit at a reduced cost, that can be applied toward the requirements for the MS in project management.
RIT’s master’s degree in project management differs from others across the country because he said RIT developed a curriculum “that is responsive to a wide range of student academic and professional needs, employs non-traditional teaching models that place a greater emphasis on project-based learning, and similar active learning experiences.” RIT’s degree also promotes strong student/faculty mentor-mentee relationships and brings project management to industries that would benefit from it but have otherwise not traditionally embraced the discipline.
The degree program allows students to customize their courses for their degrees, providing a natural path of interdisciplinary study. This allows students the ability to better specialize to their specific interests, giving them a competitive edge in their field of interest and making them more valuable to an employer.
Of the 10 courses required to earn the MS degree, four are elective, so students may use advanced certificates or other courses already offered at RIT. The remaining six classes focus on the core topics of the project management discipline and align with the standards set by the Project Management Institute, the governing body for the field.
One of those students is Dana Harp, who is taking the classes online from her home in Lewes, Del. She does clinical research remotely for Pfizer.
She received her edX project management MicroMasters in 2020 and transferred those credits toward a project management advanced certificate with RIT in 2021. She took a couple of years off from education and was pleasantly surprised when she learned RIT now offers a master’s in project management.
“I was always interested in getting my master’s degree,” Harp said. “My company has a great program to reimburse for education, so I have the opportunity to continue learning without having to pay for it all myself. And it will definitely open up more opportunities for promotion by having that degree. It will give me a leg up for the trajectory I want to be on. This is going to help me moving forward.”
Harp hopes to receive her master’s degree in the spring or next fall, and she’s excited to be one of the first students receiving the RIT degree.
“I’m lucky all of my earlier classes transferred over, and it’s really cool to see that some of the professors I’ve had in previous classes are teaching in this program as well,” she said. “I think it’s going to be really fun.”
Visit Our Website : researchdataanalysis.com
Nomination Link : researchdataanalysis.com/award-nomination
Registration Link : researchdataanalysis.com/award-registration
member ling : researchdataanalysis.com/conference-abstract-submission
Awards-Winners : researchdataanalysis.com/awards-winners
Contact us : contact@researchdataanalysis.com
Get Connected Here:
==================
Facebook : www.facebook.com/profile.php?id=61550609841317
Twitter : twitter.com/Dataanalys57236
Pinterest : in.pinterest.com/dataanalysisconference
Blog : dataanalysisconference.blogspot.com
Instagram : www.instagram.com/eleen_marissa
27 August, 2024
Discovering New DEI Strategies Through Data Analytics !
Utilizing data to conduct DEI assessments, colleges and universities can measure institutional performance and identify strategic goals to address equity gaps and disparities across campus divisions and systems. HEED Award-winning schools use data they have collected to determine the effectiveness of their innovative programs, inform decision-making, and support accountability in their institution’s DEI efforts.
Agnes Scott College utilized information collected from its annual staff survey in 2022 to achieve greater pay equity. Feedback from the survey, which included a section on retention, led the college to conduct a salary analysis for all staff and faculty. Based on those findings, 30 percent of staff received a pay adjustment and 100 percent received a retention bonus. Farmingdale State College also recently analyzed salary data campuswide. As a result, below-market salaries were increased and wages for new positions were adjusted to be more competitive.
Like many HEED schools, Georgia Southern University strives for transparency with demographic data regarding students and employees, but this school has taken the sharing of that information a step further via an enhanced web presence. The Office of Institutional Research publishes a robust, interactive dashboard of trends on their website detailing retention rates, graduation rates, faculty and staff demographics, and student population information.
In 2022, The University of Alabama at Birmingham hired a metrics analyst specifically to study its DEI-related endeavors. The position is shared equally by the Office for Institutional Effectiveness and Analysis and the Office of the Vice President for Diversity, Equity and Inclusion, and allows the school to better tell the story of its DEI efforts through analysis and interpretation of relevant data that supports such efforts.
At Kent State University, each institutional unit developed strategic planning goals for the academic year and was provided with data and feedback on their plan using newly established diversity metrics. A dynamic scorecard will show their progress at multiple benchmarking points throughout the year as they execute their programming.
Visit Our Website : researchdataanalysis.com
Nomination Link : researchdataanalysis.com/award-nomination
Registration Link : researchdataanalysis.com/award-registration
member ling : researchdataanalysis.com/conference-abstract-submission
Awards-Winners : researchdataanalysis.com/awards-winners
Contact us : contact@researchdataanalysis.com
Get Connected Here:
==================
Facebook : www.facebook.com/profile.php?id=61550609841317
Twitter : twitter.com/Dataanalys57236
Pinterest : in.pinterest.com/dataanalysisconference
Blog : dataanalysisconference.blogspot.com
Instagram : www.instagram.com/eleen_marissa
24 August, 2024
Hypotheses devised by AI could find ‘blind spots’ in research !
In early October, as the Nobel Foundation announced the recipients of this year’s Nobel prizes, a group of researchers, including a previous laureate, met in Stockholm to discuss how artificial intelligence (AI) might have an increasingly creative role in the scientific process. The workshop, led in part by Hiroaki Kitano, a biologist and chief executive of Sony AI in Tokyo, considered creating prizes for AIs and AI–human collaborations that produce world-class science. Two years earlier, Kitano proposed the Nobel Turing Challenge1: the creation of highly autonomous systems (‘AI scientists’) with the potential to make Nobel-worthy discoveries by 2050.
It’s easy to imagine that AI could perform some of the necessary steps in scientific discovery. Researchers already use it to search the literature, automate data collection, run statistical analyses and even draft parts of papers. Generating hypotheses — a task that typically requires a creative spark to ask interesting and important questions — poses a more complex challenge. For Sendhil Mullainathan, an economist at the University of Chicago Booth School of Business in Illinois, “it’s probably been the single most exhilarating kind of research I’ve ever done in my life”.
Network effects
AI systems capable of generating hypotheses go back more than four decades. In the 1980s, Don Swanson, an information scientist at the University of Chicago, pioneered literature-based discovery — a text-mining exercise that aimed to sift ‘undiscovered public knowledge’ from the scientific literature. If some research papers say that A causes B, and others that B causes C, for example, one might hypothesize that A causes C. Swanson created software called Arrowsmith that searched collections of published papers for such indirect connections and proposed, for instance, that fish oil, which reduces blood viscosity, might treat Raynaud’s syndrome, in which blood vessels narrow in response to cold. Subsequent experiments proved the hypothesis correct.
Literature-based discovery and other computational techniques can organize existing findings into ‘knowledge graphs’, networks of nodes representing, say, molecules and properties. AI can analyse these networks and propose undiscovered links between molecule nodes and property nodes. This process powers much of modern drug discovery, as well as the task of assigning functions to genes. A review article published in Nature earlier this year explores other ways in which AI has generated hypotheses, such as proposing simple formulae that can organize noisy data points and predicting how proteins will fold up. Researchers have automated hypothesis generation in particle physics, materials science, biology, chemistry and other fields.
An AI revolution is brewing in medicine. What will it look like?
One approach is to use AI to help scientists brainstorm. This is a task that large language models — AI systems trained on large amounts of text to produce new text — are well suited for, says Yolanda Gil, a computer scientist at the University of Southern California in Los Angeles who has worked on AI scientists. Language models can produce inaccurate information and present it as real, but this ‘hallucination’ isn’t necessarily bad, Mullainathan says. It signifies, he says, “‘here’s a kind of thing that looks true’. That’s exactly what a hypothesis is.”
Blind spots are where AI might prove most useful. James Evans, a sociologist at the University of Chicago, has pushed AI to make ‘alien’ hypotheses — those that a human would be unlikely to make. In a paper published earlier this year in Nature Human Behaviour4, he and his colleague Jamshid Sourati built knowledge graphs containing not just materials and properties, but also researchers. Evans and Sourati’s algorithm traversed these networks, looking for hidden shortcuts between materials and properties. The aim was to maximize the plausibility of AI-devised hypotheses being true while minimizing the chances that researchers would hit on them naturally. For instance, if scientists who are studying a particular drug are only distantly connected to those studying a disease that it might cure, then the drug’s potential would ordinarily take much longer to discover.
When Evans and Sourati fed data published up to 2001 to their AI, they found that about 30% of its predictions about drug repurposing and the electrical properties of materials had been uncovered by researchers, roughly six to ten years later. The system can be tuned to make predictions that are more likely to be correct but also less of a leap, on the basis of concurrent findings and collaborations, Evans says. But “if we’re predicting what people are going to do next year, that just feels like a scoop machine”, he adds. He’s more interested in how the technology can take science in entirely new directions.
Keep it simple
Scientific hypotheses lie on a spectrum, from the concrete and specific (‘this protein will fold up in this way’) to the abstract and general (‘gravity accelerates all objects that have mass’). Until now, AI has produced more of the former. There’s another spectrum of hypotheses, partially aligned with the first, which ranges from the uninterpretable (these thousand factors lead to this result) to the clear (a simple formula or sentence). Evans argues that if a machine makes useful predictions about individual cases — “if you get all of these particular chemicals together, boom, you get this very strange effect” — but can’t explain why those cases work, that’s a technological feat rather than science. Mullainathan makes a similar point. In some fields, the underlying principles, such as the mechanics of protein folding, are understood and scientists just want AI to solve the practical problem of running complex computations that determine how bits of proteins will move around. But in fields in which the fundamentals remain hidden, such as medicine and social science, scientists want AI to identify rules that can be applied to fresh situations, Mullainathan says.
In a paper presented in September5 at the Economics of Artificial Intelligence Conference in Toronto, Canada, Mullainathan and Jens Ludwig, an economist at the University of Chicago, described a method for AI and humans to collaboratively generate broad, clear hypotheses. In a proof of concept, they sought hypotheses related to characteristics of defendants’ faces that might influence a judge’s decision to free or detain them before trial. Given mugshots of past defendants, as well the judges’ decisions, an algorithm found that numerous subtle facial features correlated with judges’ decisions. The AI generated new mugshots with those features cranked either up or down, and human participants were asked to describe the general differences between them. Defendants likely to be freed were found to be more “well-groomed” and “heavy-faced”. Mullainathan says the method could be applied to other complex data sets, such as electrocardiograms, to find markers of an impending heart attack that doctors might not otherwise know to look for. “I love that paper,” Evans says. “That’s an interesting class of hypothesis generation.”
In science, experimentation and hypothesis generation often form an iterative cycle: a researcher asks a question, collects data and adjusts the question or asks a fresh one. Ross King, a computer scientist at Chalmers University of Technology in Gothenburg, Sweden, aims to complete this loop by building robotic systems that can perform experiments using mechanized arms6. One system, called Adam, automated experiments on microbe growth. Another, called Eve, tackled drug discovery. In one experiment, Eve helped to reveal the mechanism by which a toothpaste ingredient called triclosan can be used to fight malaria.
Robot scientists
King is now developing Genesis, a robotic system that experiments with yeast. Genesis will formulate and test hypotheses related to the biology of yeast by growing actual yeast cells in 10,000 bioreactors at a time, adjusting factors such as environmental conditions or making genome edits, and measuring characteristics such as gene expression. Conceivably, the hypotheses could involve many subtle factors, but King says they tend to involve a single gene or protein whose effects mirror those in human cells, which would make the discoveries potentially applicable in drug development. King, who is on the organizing committee of the Nobel Turing Challenge, says that these “robot scientists” have the potential to be more consistent, unbiased, cheap, efficient and transparent than humans.
Researchers see several hurdles to and opportunities for progress. AI systems that generate hypotheses often rely on machine learning, which usually requires a lot of data. Making more papers and data sets openly available would help, but scientists also need to build AI that doesn’t just operate by matching patterns but can also reason about the physical world, says Rose Yu, a computer scientist at the University of California, San Diego. Gil agrees that AI systems should not be driven only by data — they should also be guided by known laws. “That’s a very powerful way to include scientific knowledge into AI systems,” she says.
As data gathering becomes more automated, Evans predicts that automating hypothesis generation will become increasingly important. Giant telescopes and robotic labs collect more measurements than humans can handle. “We naturally have to scale up intelligent, adaptive questions”, he says, “if we don’t want to waste that capacity.”
Visit Our Website : researchdataanalysis.com
Nomination Link : researchdataanalysis.com/
Registration Link : researchdataanalysis.com/
member ling : researchdataanalysis.com/
Awards-Winners : researchdataanalysis.com/
Contact us : contact@researchdataanalysis.
Get Connected Here:
==================
Facebook : www.facebook.com/profile.php?
Twitter : twitter.com/Dataanalys57236
Pinterest : in.pinterest.com/
Blog : dataanalysisconference.
Instagram : www.instagram.com/eleen_
23 August, 2024
Sandeep Garg’s quest for data excellence !
Excellence is in the eye of the beholder, but Sandeep Garg’s contributions to the digital scapes of the cloud and artificial intelligence give rise to a unified point of view. “In technology, every milestone marks a step forward in our pursuit of excellence,” Garg says.
As one of Disney’s most dynamic data and cloud architects, he traces his two-decade journey through the high-octane landscape of software innovation.
Beginning in data
Before his position at the global entertainment conglomerate, Garg stretched his tech legs in various sectors, such as consulting, healthcare, finance, travel, and media. His software expertise has helped build and fortify the spaces of machine learning, artificial intelligence (AI), and the cloud for multiple enterprise giants in many industries.
Despite his success in different branches, one thing remained constant throughout his career. “I’ve always been a data person. I have been involved in many functions from data engineering and data analysis to data science and machine learning,” shares Garg. “From 20 years ago up to this very day, my principal goal is to explore and perhaps even redefine new aspects of data.”
Mapping out a path of innovation
Garg’s career kicked off in the bustling tech hubs of India. As a software developer in his home country, he effectively built the groundwork for a career that would soon impact the industry. From day one of his tech career, Garg was committed to excellence, which propelled him to design data platforms from the ground up.
In 2019, Garg stood on global stages, such as when he presented at Amazon Web Services (AWS) “This is my Architecture” program at the re:Invent. That same year, he spearheaded the Expedia team in migrating from on-premise operations to full AWS cloud infrastructure, speaking volumes of his proficiency in facing complex digital terrains. Proving to be a milestone-studded year for Garg, he capped it off with an Expedia Employee of the Quarter Award.
Presently working as a data and cloud architect at Disney, Garg is the mind behind numerous platforms that help Disney maintain its reputation as one of the world’s most distinguished media conglomerates.
Driving industry transformations
Garg’s dedication extends beyond conventional boundaries, as seen by his venture into data engineering, software design, architecture, analysis, and machine learning. His impact spans across sectors, from designing and developing predictive analytics models for financial institutions to revolutionizing data platforms for travel companies.
By leveraging cloud technology, Garg has also been pivotal in supporting market managers with their critical real-time decisions, thereby enhancing efficiency tenfold.
Sandeep Garg’s journey inspires aspiring technologists worldwide. His quest for data excellence demonstrates the brimming potential of human ingenuity and futuristic technology.
Visit Our Website : researchdataanalysis.com
Nomination Link : researchdataanalysis.com/
Registration Link : researchdataanalysis.com/
member ling : researchdataanalysis.com/
Awards-Winners : researchdataanalysis.com/
Contact us : contact@researchdataanalysis.
Get Connected Here:
==================
Facebook : www.facebook.com/profile.php?
Twitter : twitter.com/Dataanalys57236
Pinterest : in.pinterest.com/
Blog : dataanalysisconference.
Instagram : www.instagram.com/eleen_
22 August, 2024
Best Data Analytics Courses to Boost Your Career !
Best Data Analytics Courses to Boost Your Career
1. Google Data Analytics
This Coursera specialization has a clear learning path toward the qualification of a data analyst. The curriculum covers such classes as R programming manipulation of data, and machine learning, all done in practical assignments that would help develop relevant skills.
Key features
This specialization includes multiple courses in R programming, data analysis, and machine learning. Hands-on training will be provided in data manipulation and data visualization techniques. At the very end, it concludes with a capstone project which will apply very beneficially. A professional certificate is gained at the end, which is industry-recognized.
Course Link:Data Analytics Specialization
2. Data Analytics Crash Course
Udemy's Data Analytics Crash Course will help you realize the fastest way to achieve overall knowledge in data analytics. This course will help you learn all principal skills, from data visualization to programming languages in statistical analysis and data interpretation, to become a data analyst in the shortest possible period.
Key Features
The course will focus on real-world applications of data analytics through hands-on projects and exercises. Some of the topics covered will include lessons on data visualization tools, techniques of statistical analysis, and data cleaning. Students gain lifetime access to the course materials, and hence can flexibly learn and review.
Course Link: Data Analytics Crash Course
3. IBM Data Analyst Professional Certificate
The IBM Data Analyst Professional Certificate on Coursera will take students through the process of becoming proficient data analysts. It will offer deep, hands-on training in three of the most important tools of data analysis, Excel, SQL, and data visualization, in addition to coursework on real-world projects.
Key Features
This course includes all of the important tools and methodologies connected with data analysis, from Excel in data manipulation to SQL for database management. On top of that, it does have hands-on projects to help gain experience with real-world datasets. This course ends with a professional certificate that enables learners to enhance their career prospects.
Course Link: IBM Data Analyst Professional Certificate
4. Data Analysis with Python by IBM
As part of its course catalog, Coursera offers Data Analysis with Python from IBM, which is dedicated to teaching how to perform data analysis in Python programming language. It covers key topics, such as Python libraries used in data visualization, data wrangling, and statistical analysis. This would be a good course for those desiring to leverage Python within data analytics.
Key Features
The course enables the performance of Data Analysis with Python libraries like Pandas, Matplotlib, etc. It contains practical assignments applying the learned concepts to some real datasets. The class also contains data cleaning, visualization, and statistical analysis methods.
Course Link: Data Analysis with Python by IBM
5. Microsoft Power BI Data Analyst
This course is a comprehensive data analyst course using Power BI. It walks students through the details of using data analytics and machine learning with Power BI programming, covering real-life projects in-depth on data analysis, visualization, and machine learning algorithms.
Key Features
This course covers an in-depth study of data analysis with PowerBI, including data wrangling, visualization, and predictive modeling. It contains well-explained practical exercises and projects to build a portfolio of skills. It gives lifetime access to course content and updates.
Course Link: Microsoft Power BI Data Analyst
Conclusion
The right data analytics course can, therefore, be very important in one's career trajectory, since it aids in the acquisition of those skills necessary for excelling in this data-driven landscape. These courses presented herein shed some light on the diverse options to satisfy different learning needs, coupled with the different goals set for careers. These programs will better place you to enhance your data analytics skills and finally be successful in the job market.
Visit Our Website : researchdataanalysis.com
Nomination Link : researchdataanalysis.com/
Registration Link : researchdataanalysis.com/
member ling : researchdataanalysis.com/
Awards-Winners : researchdataanalysis.com/
Contact us : contact@researchdataanalysis.
Get Connected Here:
==================
Facebook : www.facebook.com/profile.php?
Twitter : twitter.com/Dataanalys57236
Pinterest : in.pinterest.com/
Blog : dataanalysisconference.
Instagram : www.instagram.com/eleen_
21 August, 2024
As Told To: A Finance Leader's Advice for HR in Strategic Planning !
With strategic planning coming up for many companies, I wondered how human resources leaders could enter that process as stronger and more strategic partners, so I interviewed a senior finance executive—and former management consultant—at a global public technology company. He agreed to offer frank advice on the condition he could remain anonymous—here’s what he told me:
As a finance leader, I've seen countless strategic planning cycles. HR typically comes to strategic planning meetings asking for more heads or more money for training or benefits programs. My advice to HR leaders heading into this year’s strategic planning cycle: Don’t fall into the trap of just asking for more.
Too often, HR leaders are relegated to being mere customers of the process, rather than strategic partners, meaning they’re passively receiving directives from finance and relaying them to the business. What I wish I saw from HR is a strategic perspective on what areas of the business need to grow and which could potentially shrink. They should be making decisions on the number of people that should be staffed in a particular area of the business, the structure of that area, and where more people are needed versus where we could cut back. An obvious example is understanding how AI could augment the work of roles and teams throughout the organization.
HR needs to understand what the human capital needs are that are actually going to generate positive return on investment (ROI). I don't expect HR to come up with numerical ROIs for everything they're asking for. That's probably a stretch. But if they worked with their finance partner or other business leader, together they could put together business cases for where they're asking for additional funding. This doesn't happen much in the HR world, from my experience, and it should. It’s a missed opportunity.
In addition to everyone going into these exercises asking for more resources, more heads, more money—often more than they actually need—they treat the process like a negotiation where you anchor high and hope you get what you actually want. That immediately puts you on the other side of the table from finance. We have to be the bad guy looking for ways to say no, or arbitrate and meter out the dollars or heads, which are few and far between these days.
Instead, go into that process with a two-sided approach. Show us where people are not that busy or where programs don't seem to be generating a lot of return. Identify areas where we can save or redirect investments to generate better returns or speed up high-priority projects. Know your assumptions well and ensure they are both defensible and align with the broader direction of the organization. For example, if you expect 7% attrition next year, be prepared to explain why. This balanced perspective will help you gain (or regain) credibility with the finance team.
By taking this more balanced, strategic approach, HR leaders can transform their role in the planning process. You'll shift from being seen as just another department asking for more to a true strategic partner, helping to shape the direction of the business. That's the kind of HR leadership that finance—and the entire organization—desperately needs.
HR leaders know their roles extend beyond headcount planning, and yet I’ve heard from so many who struggle to be seen as strategic, to align initiatives to business objectives, and to articulate the long-term value of investing in people and culture—all challenges I wrote about in my recent piece, “Strategic HR is at risk.” We’re sharing this finance leader’s point of view so that HR leaders who read it can begin to build closer collaborative relationships with finance—not just heading into strategic planning cycles—but throughout the year in order to develop a shared understanding of both the quantitative and qualitative aspects of human capital.
Website: International Research Data Analysis Excellence Awards
Visit Our Website : researchdataanalysis.com
Nomination Link : researchdataanalysis.com/award-nomination
Registration Link : researchdataanalysis.com/award-registration
member ling : researchdataanalysis.com/conference-abstract-submission
Awards-Winners : researchdataanalysis.com/awards-winners
Contact us : contact@researchdataanalysis.com
Get Connected Here:
==================
Facebook : www.facebook.com/profile.php?id=61550609841317
Twitter : twitter.com/Dataanalys57236
Pinterest : in.pinterest.com/dataanalysisconference
Blog : dataanalysisconference.blogspot.com
Instagram : www.instagram.com/eleen_marissa
20 August, 2024
Analysis Points to Massive Photovoltaic Deployment To Meet Decarbonization Target !
An “unprecedented ramp-up of production capacity” over the next two decades is needed to provide enough solar power to completely decarbonize the global electrical system, but that goal can be achieved, according to an analysis led by researchers at the National Renewable Energy Laboratory (NREL).
The target is 63.4 terawatts of installed nameplate capacity of photovoltaics (PV) needed in the decade between 2050 and 2060. That is a 60-fold increase in the amount of installed PV worldwide today.
Jao van de Lagemaat, director of the Chemistry and Nanoscience Center at the U.S. Department of Energy’s NREL, said a “relatively modest demand” in additional PV will be needed even after global decarbonization is reached to keep up with module retirement and population growth. This leads to an expected shock to the manufacturing industry where “suddenly much less manufacturing capacity is needed after decarbonization is achieved.”
The findings are contained in the paper, “Photovoltaic Deployment Scenarios Toward Global Decarbonization: Role of Disruptive Technologies,” which appears in the journal Solar RRL. In addition to van de Lagemaat, the other authors are Michael Woodhouse from NREL and Billy Stanbery from Colorado School of Mines.
The analysis is intended to capture the scale and temporal dynamics of the financing needed to build the manufacturing capacity to produce enough PV modules. Among the assumptions the researchers made is that after the decarbonization goal is reached, manufacturers will be reluctant to build new factories because of the drop in demand trajectories for PV modules. The factories are assumed to have a 15-year lifetime, so new ones will only be built if they are projected to sustain full output throughout their useful lifetime.
The analysis also assumes the lifespan of a PV module will increase considerably, which further exacerbates the shock to solar manufacturing because it will take longer before replacement will be needed. Researchers have been experimenting with extending the longevity of these modules from an average of 30 years in 2020 to 50 years by 2040.
To reach the decarbonization target, manufacturers will need to scale up production capacity to reach 2.9–3.7 terawatts a year within 10–15 years, a goal that will cost from $600 billion to $660 billion. The analysis shows that these goals can be reached using existing technology and using expected further cost reductions in the mature technologies that use silicon and cadmium telluride.
Disruptive solar technologies—highly efficient alternatives to the mature technologies—are going to help further lower the cost of the transition. Those technologies, such as perovskites and tandem photovoltaics that combine existing solar technologies and disruptive ones in a single much-higher-efficiency package, are forecast to be deployed at about a terawatt annually and could potentially be cheaper to manufacture than silicon PV on a per-watt basis but must be proven in the marketplace first.
In the case that these disruptive technologies can be realized, the researchers noted, cost savings for manufacturers amounting to hundreds of billions of dollars can be realized, leading to a more sustainable solar manufacturing industry.
Disruptive technologies will have an overall manufacturing market opportunity between $1 trillion and $2 trillion even if the total amount of PV installed is substantially less than 63.4 terawatts, according to the analysis, with the rest generated by other sources of renewable or carbon-free energy such as wind and nuclear.
“There are economically viable trajectories that get to the needed manufacturing capacity to produce the amount of PV needed to completely decarbonize the world’s energy economy,” van de Lagemaat said. “Emerging technologies could potentially lower the cost of this deployment significantly if they get commercialized in time.”
NREL’s internal Laboratory Directed Research and Development program funded the research.
Visit Our Website : researchdataanalysis.com
Nomination Link : researchdataanalysis.com/
Registration Link : researchdataanalysis.com/
member ling : researchdataanalysis.com/
Awards-Winners : researchdataanalysis.com/
Contact us : contact@researchdataanalysis.
Get Connected Here:
==================
Facebook : www.facebook.com/profile.php?
Twitter : twitter.com/Dataanalys57236
Pinterest : in.pinterest.com/
Blog : dataanalysisconference.
Instagram : www.instagram.com/eleen_
19 August, 2024
Impact of Geospatial Analytics on Predictive Capabilities !
Understanding Geospatial Analytics
Geospatial analytics primarily focuses on collecting, presenting, and altering data related to images, GPS, satellite images, and records, all of which are geographically linked. This method of analysis enables the presentation of data in a manner that highlights connections, patterns, and trends through the use of maps, globes, reports, and graphs.
Enhancing Predictive Capabilities
Predictive modeling is entering a new phase with the integration of artificial intelligence (AI) and geographic analysis. This inventive approach, Geospatial Analytics on Predictive Capabilities, investigates the area of forecasting future events rather than only identifying places. Take the example of urbanization. Within this area, the movement of vehicles, the process of enrolling students in schools, and the demand for emergency services are among the components of urban living that geospatial analysis can forecast the effects of new housing developments on. For those engaged in urban planning, this ability to forecast offers a significant improvement, as it enables them to take actions that influence the expansion of metropolitan areas.
Applications Across Industries
The predictive value of geospatial analytics is multifaceted. It aids in forecasting crop yields and insect infestations in agriculture. It is used in environmental conservation to predict how wildfires will spread and how climate change will affect specific areas. The retail industry uses it to determine the best locations for new stores based on consumer behavior patterns.
Challenges and Considerations
Geospatial analytics provides numerous advantages, but it's essential to be aware of the obstacles involved. Protecting data privacy and security is of utmost importance, given that geospatial data can be pretty sensitive. Furthermore, the quality of the data and the computational techniques used determine how reliable the forecasts are.
The Future of Geospatial Analytics
Advancements in technology are propelling geospatial analytics into a promising future. The capability to process geospatial data accurately and in real-time is sharpening our predictive tools.
Geospatial analytics is completely transforming our approaches to strategic planning and data analysis and has a considerable impact on the capacity for outcome prediction. As long as we continue harnessing Geospatial Analytics on Predictive Capabilities potential, innovative applications will emerge, gradually changing industries and improving our understanding of the world around us.
Geospatial Analytics
Geospatial Analytics on Predictive Capabilities
Predictive Capabilities
Geospatial Data Analytics
Website: International Research Data Analysis Excellence Awards
Visit Our Website : researchdataanalysis.com
Nomination Link : researchdataanalysis.com/award-nomination
Registration Link : researchdataanalysis.com/award-registration
member ling : researchdataanalysis.com/conference-abstract-submission
Awards-Winners : researchdataanalysis.com/awards-winners
Contact us : contact@researchdataanalysis.com
Get Connected Here:
==================
Facebook : www.facebook.com/profile.php?id=61550609841317
Twitter : twitter.com/Dataanalys57236
Pinterest : in.pinterest.com/dataanalysisconference
Blog : dataanalysisconference.blogspot.com
Instagram : www.instagram.com/eleen_marissa
16 August, 2024
Saudi Arabia’s Tourism Sector Set For Transformation With New Data Lab From Visa And STA !

Saudi Arabia’s tourism sector is poised for transformation as Visa and STA launch a groundbreaking Data Lab to enhance visitor experiences and drive industry growth.
In a groundbreaking initiative for the region, Visa, a global leader in digital payments, has partnered with the Saudi Tourism Authority (STA) to launch the Tourism Data & Campaigns Management Lab. This innovative Lab is designed to align with the Saudi government’s vision to elevate the nation’s tourism industry and enrich the overall visitor experience.
The Visa-STA Tourism Data Lab leverages cutting-edge data analytics to provide valuable insights into travel and tourism trends. These insights empower STA to make strategic decisions regarding tourism campaigns and initiatives, fostering greater collaboration with stakeholders in the travel industry.
Driven by VisaNet, the world’s most extensive payments network, this collaboration offers a robust dataset that includes detailed information on visitor journeys, spending patterns, seasonality, digital adoption, and customer demographics. The data equips the Saudi government with the tools to optimize cashless strategies and deliver more personalized, seamless payment experiences for tourists.
Saudi Arabia, officially known as the Kingdom of Saudi Arabia (KSA), is a prominent country in West Asia and the Middle East. Occupying the majority of the Arabian Peninsula, it spans approximately 2,150,000 square kilometers (830,000 square miles), making it the largest nation in the Middle East and the fifth-largest in Asia. The country is bordered by the Red Sea to the west, Jordan, Iraq, and Kuwait to the north, the Persian Gulf, Bahrain, Qatar, and the United Arab Emirates tothe east, Oman to the southeast, and Yemen to the south. The Gulf of Aqaba in the northwest creates a natural separation between Saudi Arabia and Egypt and Israel. Uniquely, Saudi Arabia is the only nation with coastlines on both the Red Sea and the Persian Gulf. The landscape is predominantly characterized by arid deserts, lowlands, steppes, and mountainous regions. Riyadh serves as the capital and largest city, with Jeddah and the Islamic holy cities of Mecca and Medina also being major urban centers. With nearly 32.2 million inhabitants, Saudi Arabia ranks as the fourth most populous country in the Arab world.
Website: International Research Data Analysis Excellence Awards
Visit Our Website : researchdataanalysis.com
Nomination Link : researchdataanalysis.com/award-nomination
Registration Link : researchdataanalysis.com/award-registration
member ling : researchdataanalysis.com/conference-abstract-submission
Awards-Winners : researchdataanalysis.com/awards-winners
Contact us : contact@researchdataanalysis.com
Get Connected Here:
==================
Facebook : www.facebook.com/profile.php?id=61550609841317
Twitter : twitter.com/Dataanalys57236
Pinterest : in.pinterest.com/dataanalysisconference
Blog : dataanalysisconference.blogspot.com
Instagram : www.instagram.com/eleen_marissa
14 August, 2024
statistical software !
Statistical software refers to computer applications designed to perform statistical analysis and data management. These tools are essential for researchers, analysts, and data scientists across various disciplines, providing powerful capabilities to manipulate, analyze, visualize, and interpret data. Statistical software typically includes features for:
- Data Management: Importing, exporting, and cleaning datasets, as well as handling large datasets.
- Statistical Analysis: Performing descriptive statistics, inferential statistics, regression analysis, hypothesis testing, and more complex modeling techniques.
- Data Visualization: Creating graphs, charts, and other visual representations of data to aid in interpretation and presentation.
- Predictive Modeling: Building models to forecast outcomes based on historical data, including machine learning algorithms.
- Reporting and Documentation: Generating outputs in various formats (e.g., tables, reports) for publication or further analysis.
Some popular statistical software packages include:
- R: An open-source language and environment specifically designed for statistical computing and graphics.
- Python (with libraries like pandas, NumPy, and SciPy): A versatile programming language that, with the right libraries, is powerful for statistical analysis.
- SPSS (Statistical Package for the Social Sciences): A widely used software for data analysis in the social sciences.
- SAS: A comprehensive suite used for advanced analytics, business intelligence, and data management.
- Stata: Known for its user-friendly interface and capabilities in econometrics, biostatistics, and epidemiology.
- Minitab: Frequently used in quality management and process improvement for its easy-to-use statistical analysis tools.
- MATLAB: While primarily a numerical computing environment, it offers robust tools for statistical analysis.
- JMP: Developed by SAS, it focuses on exploratory data analysis and visualization, often used in engineering and research.
These tools support a range of applications from academic research to industrial processes, enabling users to extract meaningful insights from data.
Website: International Research Data Analysis Excellence AwardsAwards-Winners : researchdataanalysis.com/awards-winners
Get Connected Here:
==================
Facebook : www.facebook.com/profile.php?
Twitter : twitter.com/Dataanalys57236
Pinterest : in.pinterest.com/
Blog : dataanalysisconference.
Instagram : www.instagram.com/eleen_
DeepSeek research suggests Huawei's Ascend 910C delivers 60% of Nvidia H100 inference performance !!!
Huawei's HiSilicon Ascend 910C is a version of the company's Ascend 910 processor for AI training introduced in 2019. By now, the p...
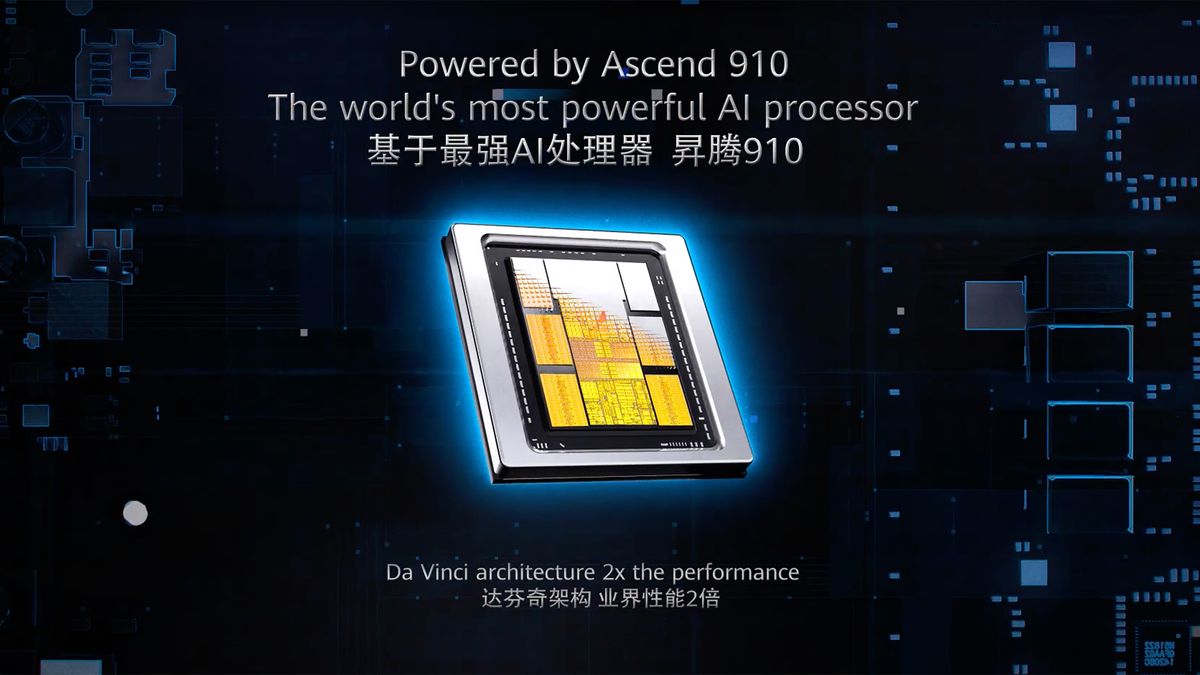
-
SDG Group USA has unveiled its "2025 Data, Analytics & AI Trends," highlighting 12 key developments poised to shape the futu...
-
Global Open Data Initiatives Highlight recent government or institutional policies promoting open access to data, such as new regulations...
-
High school students who are pursuing a degree in a STEM field usually focus on their coursework and SATs with the hope of getting accepte...